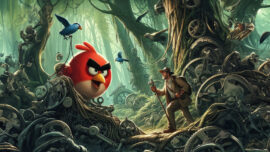
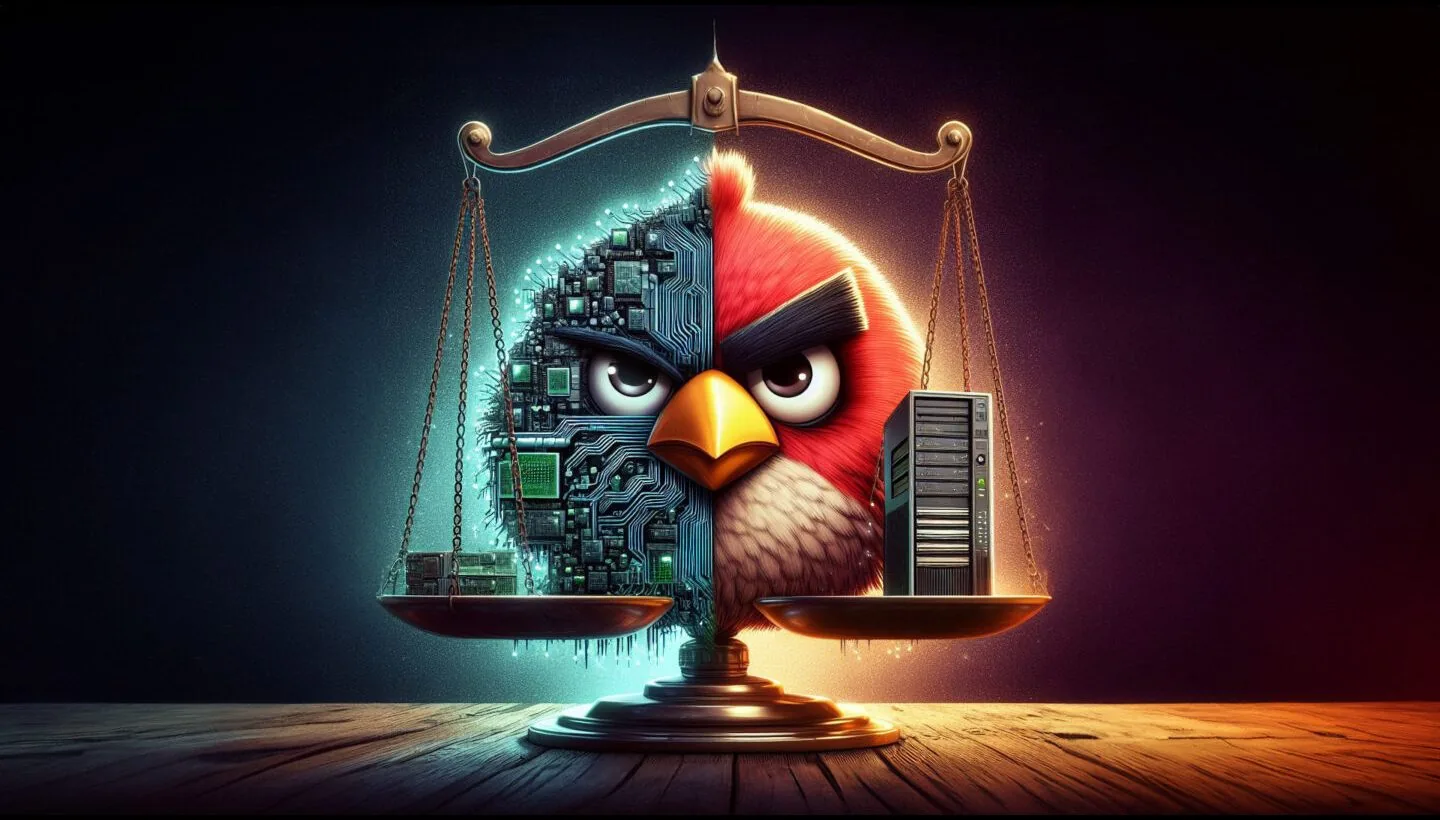
An analysis on the troubling use of AI in people focused systems from Rovio’s DEI People Analytics Lead and Machine Learning Engineer, Bee Steer.
Written by: Bee Steer, DEI People Analytics Lead, Machine Learning Engineer
If you were applying for a job at Amazon back in 2015, you ought to pick up the sport lacrosse and legally change your name to Jared to dramatically increase your chances of being hired, at least theoretically. Now the question is, why were these qualities seen as desirable by Amazon? In truth, they weren’t really. Amazon had made the mistake of implementing AI into their recruitment processes that was trained with internal data, which had an above average number of men called Jared, and also people who played lacrosse. Not only that, but as is the case with the tech industry, there was an over representation of men in general, which led to the AI throwing out almost all female candidates. The CVs the AI were told to process were still anonymised with regard to name and gender, but neural nets are smart (or dumb) black boxes after all. It learnt that any candidate who proudly displayed something non-men oriented, for example “Organised a women in tech event” or “Captain of women’s sport team”, was filtered out.
Luckily the issues in the system were quickly realised, and Amazon claims was never actually used to evaluate a candidate. However, if we imagine a world where recruitment AI was left unchecked we might be living in a Brave New World-esque society where all tech workers were lacrosse playing men named Jared. Realistically though, we’d likely see the recent upwards trends and progress in hiring diversity into tech decline dramatically. This is why unveiling biases in AI is so important, and why I believe it is our duty to be questioning these systems throughout the entire model development process.
Some More Case Studies
Despite what recent trends may have you believe, AI and machine learning have been around for a long time, with many mistakes being made, which gives us a lot of great insight into what not to do when designing algorithms with people in mind. Below is nowhere near an exhaustive list, but a selection that covers some of the more significant or interesting examples.
Radicalisation from YouTube Engagement
Back in 2019, YouTube updated their recommendation systems in response to a particular ‘quirk’ in their ML algorithm. They had chosen to recommend new and popular videos to people based on raw engagement metrics like how many times a video had been watched overall. This makes sense at face value, but the issue with this somewhat minimalist approach is that when you have a small but extremely engaged group of users watching videos, the general engagement of said videos is very high.
This was exactly the case with conspiracy theory content on YouTube. A fervent audience for conspiracy related content boosted engagement numbers, which resulted in the content being recommended to more users, and also incentivised content creators to make more videos, and so the cycle continued. These algorithms had essentially optimised themselves to draw impressionable people into a radical conspiracy mentality. To correct this phenomenon, YouTube had to specifically start filtering out flat earth and QAnon videos from being recommended by reworking their whole system.
This tendency to favour certain content over others, even if it means boosting extremist views, highlights the bigger problem with AI. Unchecked algorithms can potentially give huge platforms to hateful messages and radicalise vulnerable people. When platforms like YouTube have users viewing roughly 1 billion hours of video total per day, social media has a massive societal influence. Mixing that with a naive AI could spell disaster without the ethical and conscious engineering needed of such systems.
Gender Biases and Google Translate
When it comes to machine translating fairly gender-neutral languages like Finnish, Estonian and Hungarian, Google Translate and similar tools sometimes hit a dangerous stumbling block. These languages don’t have gendered pronouns, so the translations end up relying on some form of context to gender the sentence. The catch is, since models like Google Translate are trained on huge sets of text data, many gender and cultural biases echo through. Neutral statements like the Finnish “Hän on insinööri” get translated into English as “He is an engineer”, as opposed to “They are an engineer”. Although we’re straying away from machine learning and into the field of translation (which in itself is an art), I’d like to point out that singular ‘they’ in the English language is not only grammatically correct but has been used since the 14th century so is also a good translation for the Finnish neutral “Hän”.
Hungarian to English translation via Google Translate (retreived in 2021):
Hungarian:
Ő szép. Ő okos. Ő olvas. Ő mosogat. Ő épít. Ő varr. Ő tanít. Ő főz. Ő kutat. Ő gyereket nevel. Ő zenél. Ő takarító. Ő politikus. Ő sok pénzt keres. Ő süteményt süt. Ő professzor. Ő asszisztens.
English translation:
She is beautiful. He is clever. He reads. She washes the dishes. He builds. She sews. He teaches. She cooks. He’s researching. She is raising a child. He plays music. She’s a cleaner. He is a politician. He makes a lot of money. She is baking a cake. He’s a professor. She’s an assistant.
Issues like this no longer exist in at least some languages in Google Translate since this was realised and a fix first rolled out back in 2018, (yet, the above example is from 2021). However, this problem still exists ‘under the hood’ of the translations in the training data set. These biases are clearly damaging, and it’s quite easy to think about how the grand scale of such gender assumptions outside of translation may affect people, especially in a time where under-representation of women in STEM is still a major issue, and general gender inequalities in the workplace and home are still worse than before the covid pandemic.
Feminine Presenting Erasure Through Content Filtering
Most, if not all, social media adopts a level of content filtering to remove what’s deemed unsafe content, such as images of violence or nudity for example. With nearly 100 million images uploaded to instagram every day (as of 2023), the use of AI can be vital in flagging such content to protect its audience. An issue was highlighted in 2023, when a Guardian investigation discovered that these same algorithms are much more likely to rate female presenting bodies as more ‘racy’ and to censor the content more often, compared to very similar content with male presenting bodies. Not only is this demonstrating a level of manufactured objectification of women but it also furthers erasure of women’s voices through erasing their content. Especially with the rise of social media marketing and influencers, a social media presence can be important for one’s livelihood, but the investigation highlighted content creators getting ‘shadowbanned’ and having drastically less views for the same written content, depending on if there was an attached image of women or not. There’s also direct health risks as the tested models from Microsoft, Amazon and Google were all labelling medical pictures such as a US National Cancer Institute clinical breast examination or pictures of pregnant people as explicitly sexual in some way.
Since these algorithms exist as a black box, hidden away under the layers of social media, there’s a severe lack of transparency with how the systems operate and act. Women and other gender minorities are coaxed into ‘covering up’ more than men or risk being made invisible through shadowbanning or filtering, leading to less expression and freedom for marginalised groups online.
Concerns with Generative Art
Generative AI, as implied by its name, encompasses various forms of AI designed to produce content, such as images, text and sound. It is one of the most talked about forms of machine learning and has probably become the most quickly adopted phenomena of all time after OpenAI’s ChatGPT reached 100 million users just 2 months after its launch. This enthusiasm triggered a competitive atmosphere, evident in the swift release of subsequent models like GPT-4 only a few months after ChatGPT. The field of image generation AI also experienced a surge in popularity, exemplified by OpenAI’s DALL-E Mini in November 2021, followed by DALL-E 2 a few months later again. Today, many other image generation tools are widely used, such as Stable Diffusion, Midjourney, Adobe Firefly, and now DALL-E 3. Here we emphasise the quick pace of model development as it demonstrates the need to address and quantify the extent of any inherent biases, while also highlighting the potential cut corners associated with the speed of sourcing larger and larger datasets for model training.
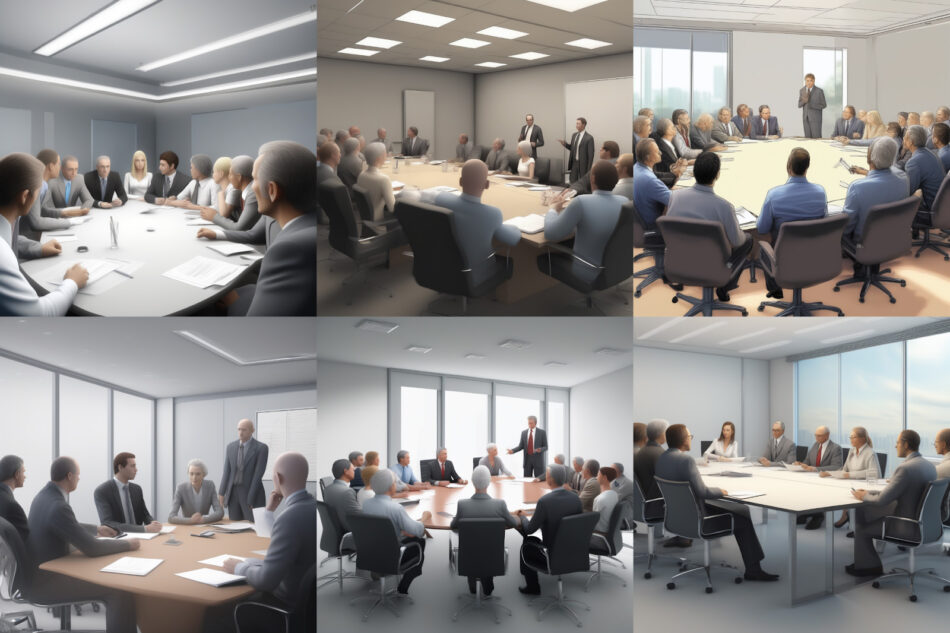
Recently Jorge Ramírez Carrasco and I did a talk at RovioCon Google 2023 explaining how we use generative AI in the design process for Angry Birds 2. These ethical issues were addressed with the following example; the figure here was generated using a generative art model with the prompt “people in a very important meeting”. We see that, although clearly a meeting is taking place, there seems to be an over representation of a particular demographic, specifically what looks like older Caucasian men. Here it seems pretty clear the major risks of generative AI exacerbating racist and sexist views, and in fact it’s such an obvious problem that even OpenAI has openly expressed and documented these issues with Dall-E. At least here at Rovio, we’re quite proud that this is quite far off representative of how our ‘very important meetings’ look and although these systems seem to be full of risks against our DEI efforts in the workplace there are many ways in which we are able to combat this to take full advantage of new AI tools.
These are just a few examples that underscore the critical importance of addressing biases in artificial intelligence systems. From flawed hiring algorithms favouring irrelevant qualities in applicants, to YouTube’s unintentional radicalization, and from gender biases in translation algorithms to social media content filtering perpetuating gender inequalities, these instances emphasise the potential societal repercussions of unchecked AI systems. As we delve into various case studies, it becomes evident that biases in AI can lead to significant ethical concerns, shaping and reinforcing harmful narratives. Part 2 of this blog will explore potential solutions and strategies to mitigate biases in AI, as well as some details about how AI is used at Rovio, aiming to contribute to the ongoing dialogue surrounding responsible and ethical AI development. Stay tuned for actionable insights into creating more inclusive and fair artificial intelligence systems.